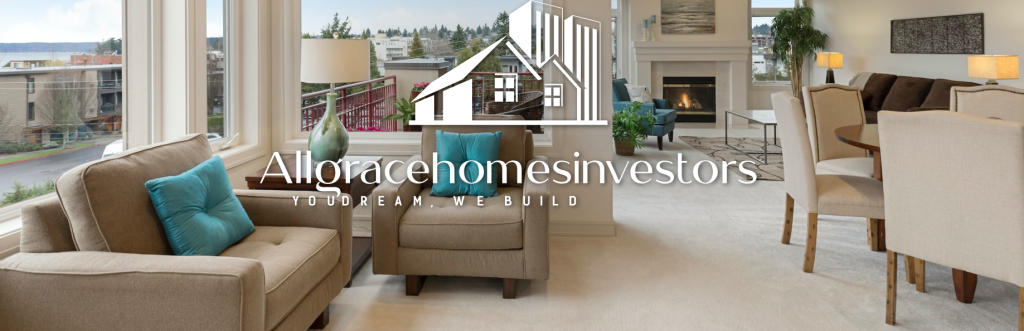
Home prices are expected to rise by about 5% in 2023, and then remain relatively stable after that. The number of millennials in the market is expected to grow by about 4% per year over the next few years. The use of predictive analytics is expected to grow by about 15% per year. The exact statistics vary depending on the source and the specific trend.
Predictive analytics uses data and algorithms to make predictions about future trends. In real estate, this can be used to predict things like home prices, rental rates, and demand. It can also be used to help find the best location for a new development or to identify properties that are at risk of foreclosure. Predictive analytics is becoming more widely used in the real estate industry as the amount of data available increases.
seven steps to creating predictive messages in real estate:
- 1. Define your goals.
- 2. Gather data.
- 3. Clean and analyze the data.
- 4. Develop your models.
- 5. Test and evaluate your models.
- 6. Optimize your models.
- 7. Deploy your models.
DEFINE YOUR GOALS
Defining your goals. Before you can create predictive messages, you need to know what you want to achieve. This could be something like increasing sales, reducing marketing costs, or finding new customers. Knowing your goals will help you to focus your efforts and make the most of your data.
GATHER DATA
Gathering data. To create predictive messages, you need access to relevant data. This could include data on previous sales, demographics, market trends, and customer behavior. The more data you have, the more accurate your models will be. There are three main types of data used in predictive analytics: historical data, real-time data, and internal data. Historical data is data from the past, such as sales trends or customer behavior. Real-time data is data that is updated in real time, such as current market trends. Internal data is data that comes from within your organization, such as sales figures or marketing campaigns.
CLEANING AND ANALYZING THE DATA
Cleaning and analyzing the data. Once you have collected the data, it needs to be cleaned and analyzed before it can be used for predictive analytics. This involves removing any errors or duplicates, and organizing the data into a format that can be used for modeling. Data cleaning and analysis is a very important step, as it can have a big impact on the accuracy of your models.
DEVELOPING THE MODELS.
Developing the models. Once the data is cleaned and organized, it can be used to develop predictive models. These models use algorithms to analyze the data and make predictions about future events. There are many different types of predictive models, such as regression models and neural Networks. Regression models use historical data to predict future values of a variable. For example, a regression model could be used to predict how sales will change in response to changes in marketing spend. Neural networks are a type of machine learning algorithm that can identify complex patterns in data. They are often used for tasks like image recognition and language processing.
TESTING AND EVALUATING THE MODELS
Testing and evaluating the models. After the models have been developed, it’s important to test them on new data to make sure they are accurate. This is called model validation, and it’s a critical step in the process. Models can also be evaluated using metrics like accuracy, precision, and recall.
OPTIMIZING THE MODELS
Optimizing the models. Even after a model has been validated, it can still be improved. This process is called optimization, and it involves fine-tuning the model to get the best possible results. There are many different ways to optimize a model, and the specific approach will depend on the type of model and the data. Hyperparameter tuning and regularization. Hyperparameter tuning is a method of finding the best values for certain parameters in the model. Regularization is a technique that helps to prevent overfitting, which is when a model performs well on the training data but not on new data.
DEPLOYMENT
Deployment. Once a model has been optimized, it can be deployed to a production environment, where it can be used to make predictions in real time. Deployment can be done in many different ways, such as on a server, in the cloud, or even on a mobile device.
The advantages of predictive analytics in real estate. First,
- It can help to identify trends that would otherwise be difficult to see. For example, it can help to identify hot spots for development or to predict changes in property values.
- It can help to make better decisions about pricing and marketing. By analyzing data on previous sales, it’s possible to find the optimal price point for a property.
- It can help to find the best location for a new development. By analyzing data on factors like crime rates, access to amenities, and proximity to major employers, it’s possible to identify the most promising locations.
- It can help to reduce risk. By identifying properties that are at risk of foreclosure or vacancy, it’s possible to take steps to prevent financial loss.
There are a few potential disadvantages of predictive analytics. One is
- The risk of bias. If the data used to train the model is biased, then the model itself may be biased as well.
- Another disadvantage is the potential for misuse of the model. If the model is not properly understood or if it is used for unethical purposes, it can cause harm. Finally,
- There is the risk of over-reliance on the model. If people become too reliant on the predictions made by the model, they may ignore other sources of information.
- The potential for privacy concerns. If sensitive data is used to train the model, there is a risk that the privacy of individuals could be compromised.
- the possibility of black box models. These are models that are so complex that they are difficult to understand, which can make it difficult to explain or correct errors.
- Do you want to hear about any other.
In real estate, predictive analytics is used in a variety of ways. One example is
- Predictive maintenance, where sensors are used to monitor the condition of buildings and alert owners when repairs are needed.
- Predictive modeling of market trends, which helps real estate agents to make better decisions about pricing and marketing.
- Predictive analytics is used in the mortgage industry to assess credit risk and make lending decisions.
- Property valuation: Predicting the value of a property based on factors such as location, size, condition, and amenities.
- Property management: Predicting when maintenance or repairs will be needed, and optimizing the timing of these tasks.
- Lead generation: Predicting which leads are most likely to convert into sales.
- Marketing: Personalizing and targeting marketing campaigns based on individual preferences and behavior.
- Predictive analytics can be very powerful, but it’s important to consider both the advantages and disadvantages.
- Bias and privacy are two of the biggest risks associated with predictive analytics.
- It’s important to properly train and maintain models, and to avoid over-reliance on them.
- There are many different ways to use and deploy predictive analytics models.
- The cost and complexity of predictive analytics should be considered.
Predictive analytics is a powerful tool that is being used in many industries, including real estate. It can help organizations to make better decisions, improve efficiency, and create more personalized experiences. However, it’s important to consider the potential risks and limitations of this technology.
0 Comments